Core Concepts of Predictive Marketing
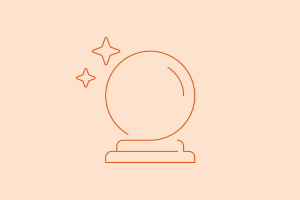
We believe that anyone can learn to do predictive marketing with the right foundation. Excerpts from Predictive Marketing: Easy Ways Every Marketer Can Use Customer Analytics and Big Data, written by Acquia’s former Chief Science Officer Omer Artun, provide that baseline. Read on to learn about relationship marketing and predictive analytics in marketing.
1. Predictive marketing use cases
Improve precision of targeting and acquisition efforts. With predictive marketing, it is possible to know which channels produce the most profitable customers and to optimize marketing spending based on this knowledge. Armed with better information about behavioral buying personas, marketers can also design more effective acquisition campaigns that hypertarget a specific microsegment and increase conversions by four times or more.
Use personalized experiences to increase lifetime value. Predictive marketing can predict future customer preferences and interactions (such as a customer’s likelihood to buy). Armed with this information, marketers can improve personalization, relevancy, and timing of customer interactions. It is these experiences that will keep customers coming back and maximize customer lifetime value. If you can maximize the lifetime value of each of your customers, you will automatically maximize the value of your entire customer portfolio and the value of your company as a whole.
Understand customer retention and loyalty. Predicting when, why, and which customers will return or leave is a big challenge for many organizations. Predictive marketing can help flag customers who are at risk of leaving so that marketers can take proactive steps to retain these customers. Predictive analytics can also generate insights about loyalty-inducing behaviors that maximize customer lifetime value.
Optimizing customer engagement. Predicting who will respond to an email promotion, what would it take to convert a browser into a buyer, and what discount is needed to incentivize the customer to complete the transaction are all methods of increasing customer engagement in real time or near-real time that maximizes marketing effectiveness.
10 examples of predictive marketing
10 Questions to Answer |
How Predictive Marketing Can Help |
Who will your best customers be? |
Predict which prospects or customers have the highest lifetime value, taking into account revenues, but also the cost to acquire and service these accounts. Use this information to spend time and money on high potential customers early on. |
How can you find more new customers like your existing best customers? |
Predict which prospects are most like your existing high-value customers using look-alike targeting (B2C) or specialized lead generation vendors (B2B). |
Find personas in your data to use to acquire more customers like this. |
Predict the customer clusters that most distinguish buying personas with respect to brands, products, content, and behaviors in your customer base. Then develop creative, content, products, and services to attract more buyers like this. |
Which marketing channels are most profitable? |
Predict which channels attract the customers with the highest lifetime value, including all future purchases. Use this information to influence keyword bidding strategies and channel investments. |
Which prospects (non buyers) are most likely to buy? |
Determine who is most likely to buy so you can give the right incentive (in B2C) or prioritize your sales personnel’s time with the right prospects (in B2B). |
Which existing (or past) customers are most likely to buy? |
Product incentive (or discount) is needed to convince a one-time buyer to become a repeat customer. Prioritize the time of account managers to focus on likely upsell candidates. |
Which existing customers are least likely to buy? |
Predict which customers are likely to leave and target them proactively with a “please come back” incentive, a personalized recommendation, or by having the customer success manager make a call. |
What customers might be interested in a specific new product? |
Predict which customers might be interested in overstock items or a new product release so you can focus your sales and marketing efforts on these businesses or consumers. |
What other products or content might this customer be interested in? |
Predict what product or content recommendations to make to a particular customer in order to win, upsell, or re-engage this customer. |
What is my share of wallet with a specific customer? |
Predict what markets or customer groups have high value potential to focus future customer acquisition strategies. |
Armed with information ranging from likelihood to buy, predicted lifetime value, and future product preferences, brands can better serve their prospects and their customers by delivering personalized experiences. Applying machine learning and predictive analytics to marketing takes the guesswork out of knowing your customer preferences so you can leave the right and strongest impression on your customers.
2. Developing a predictive marketing strategy
Thus far we’ve gone over predictive marketing use cases. Now it’s time to get things started. You don’t have to do everything at once. Just get started.
Choosing the wrong vendor or the wrong campaign is not as bad as waiting. Your competitors are already leveraging predictive marketing today and gaining a significant competitive advantage from their early experiments. Remember that many companies are seeing the lifetime value, retention, and loyalty of their customers increase dramatically using predictive marketing techniques. Here are three recommendations.
Get started small
With a couple of thousand dollars a month and a couple of weeks of integration work, you can begin solving your customer data problem and run your first marketing campaign. The best way to build a case for predictive marketing is to just get started. Given the large returns expected for this investment, you really cannot afford to wait. Ask yourself how you would feel if your competitors deployed this type of technology first. How would your customers feel if they are getting personalized treatment from your competitors first but not from you? Also ask yourself if there are other projects on your plate that can truly give you a higher return on investment.
Bring customer data in house but outsource the data science
We strongly believe that it is not possible to become truly customer-centric without making customer data available to all customer-facing personnel in your organization, starting with you — the marketers. Therefore, we strongly recommend against outsourcing your customer database to a third-party provider such as a marketing service provider. It will be too difficult to access data when and how you want it, and the customer insights will reside outside your organization.
Bringing customer data in-house does not mean that you need to hire expensive data scientists or technology resources. Easy-to-use, online solutions are available that allow you to own and access your customer data at any time, but use outside vendors to create the advanced statistical models. Data scientists are in high demand, and most marketers do not have the bandwidth and expertise to hire, provide direction, and retain such analytical personnel. The best data scientists that truly make an impact are the ones that have business acumen, which are even harder to find. Data science is a good way to gain insights, but it is hard to make the information available at every customer touchpoint without extensive IT projects. Therefore, start with the end in mind and find the most practical solution that gets you to making a difference in the way your customers interact with your brand.
Complement your existing infrastructure with predictive marketing
You don’t have to rip and replace your existing infrastructure. You can certainly get started by complementing your existing infrastructure with robust data cleansing and predictive capabilities. Start small and expand your deployment over time. This could include embedding predictive capabilities into your different marketing channels, such as email, and perhaps replacing your existing specialized tools with a single campaign management platform to coordinate campaigns across all channels.
3. Using look-alike targeting
Remarketing only works for known visitors. Remarketing can help you convert more browsers into buyers and get past buyers to come back and buy again. However, remarketing cannot help you find and acquire new consumers for your products, services, or content. This is where look-alike targeting comes in.
Look-alike targeting is a predictive analytics technique to find people who look just like an initial “seed audience.” For example, if you feed a look-alike targeting system — such as Facebook’s look-alike audiences — a list of your existing customers, it can find prospects that have the same characteristics as your existing customers. You can now use this “look-alike audience” to launch an advertising campaign.
Figure 1 illustrates the concept, using Facebook as an example. Facebook look-alike targeting is increasingly popular, but Facebook is not the only network that gives advertisers the ability to use look-alike audiences. Many advertisers, including Twitter, Google Display Network, and others also offer look-alike audience capabilities.
On Facebook, you start by uploading a specific list of customers to Facebook custom audiences. This can be the list of customers who prefer leather products or perhaps the list of your best customers. Facebook will now try to match these records to its user database. Matching happens based on email address. The list needs to contain at least 100 records that match to a Facebook account. After matching at least 100 users, Facebook now uses their internal algorithms, which also use predictive analytics, to go out and match your segment to other new people in the Facebook database that “look like” your original list.
Figure 1: Facebook look-alike targeting
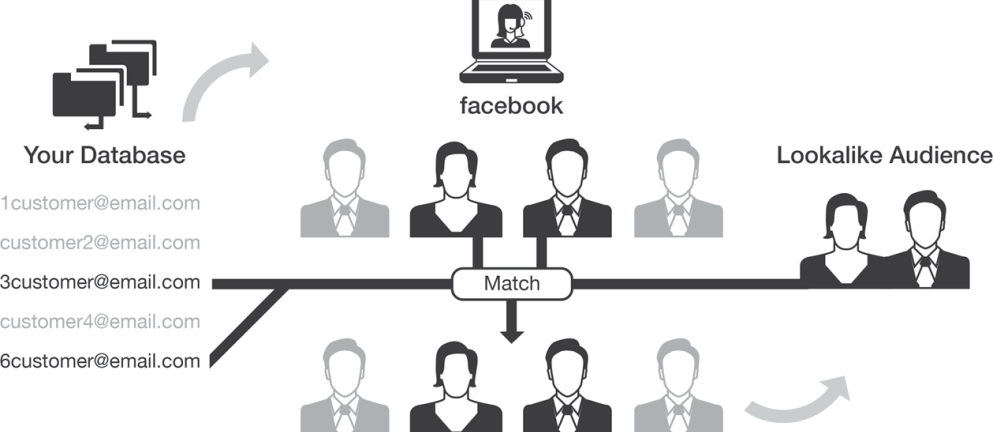
Look-alike modeling is a powerful tool that enables marketers to go out and target people who have similar traits or behaviors to their existing customers or website visitors. Look-alike algorithms typically need to be fed a list of at least 100 or more existing visitors or customers as a “seed.”
Look-alike audiences can be used to support any business objective: targeting people who are similar to sets of customers for fan acquisition, site registration, purchases, and coupon claims, or simply to drive awareness of a brand. It can also be used to find audiences who put an item in the basket of your website but didn’t pay for it.
Before using look-alike targeting, make sure your seed audience is clean and well selected or else the look-alike targeting algorithms will not work. Look-alike targeting is only as good as the inputs are. Remember, here, too: garbage in, garbage out. Make sure your seed audience converts really effectively before you expand the seed audience with look-alike targeting. Go for quality before quantity for your seed audience. We recommend that you start with a look-alike campaign that is based on your best customers. These are customers that have bought from you several times, and therefore you are sure that these are quality customers.
Optimizing for similarity or reach
Marketers can optimize their look-alike campaigns for “similarity” or “reach.” When optimizing for similarity, marketers are looking for impressions with tight accuracy — and presumably better results.
You could say, for example, “with 90 percent certainty, I know that this person will buy from you.”
There will be fewer of these customers than customers who have, say, a 60 percent chance to buy from you. When optimizing for “reach,” the match is hazier and the ROI lower, but you might acquire more customers overall.
On Facebook, you can choose to optimize your audience for “similarity” or “reach” automatically or to customize something in between. When optimized for similarity, a look-alike audience will include the top 1 percent of people in the selected country who are most similar to the seed custom audience. The reach of the new audience will be smaller, but the match will be more precise.
When optimized for reach, a look-alike audience will include the top 5 percent of people in the selected country that are similar to the seed custom audience but with a less precise match.
Instead of using the types (explained earlier), you can manually set a ratio value that represents the top X percent of the audience in the selected country. The ratio value should be between 1 percent and 20 percent and should be specified in intervals of 1 percent.
A North American beauty company used product-based clusters to launch specific look-alike advertising campaigns on Facebook. They first uploaded a list of all existing customers who were part of a bath and body cluster. Then they designed the creative for a Facebook advertising campaign specifically to appeal to this type of bath and body customer. This combination of clustering and look-alike targeting turned out to be highly profitable. For the North American beauty company, these campaigns delivered between 2 and 10 times return, when comparing revenues generated to the investment made in Facebook ads.
Look-alike targeting works on many other advertising networks, not just Facebook. The mechanisms for selection and purchasing media are similar across networks and new options become available every year.
4. Predicting individual recommendations for each customer
A North American beauty and cosmetics company with hundreds of stores was looking to personalize its communications to hundreds of thousands of customers and ensure that every online interaction between the brand and its customers was consistent with its messaging. It wanted to shift the mindset from being discount-driven to improving customer service and satisfaction.
The company chose to combine cluster-based targeting with personalized recommendations to send customers more strategic, personalized offers. The company first used predictive analytics to organize its customers in product-based clusters such as “bath and beauty” and “face cream.” Then it emailed each of these customers content and recommendations that were based on their cluster. Customers liked the emails, and the company was able to increase revenues per email by six times.
Recommender systems have been around for almost 20 years, Amazon being the primary example that started using this early on. There are three parts to making personalized recommendations: sending recommendations to customers at the right time, understanding the context, and sending the right content.
First-generation recommender systems used simple rules configured by human beings based on things like keywords or titles. In other words, a merchandiser or content marketer set up a rule so that everybody who bought shoes would get a recommendation for protective spray shortly thereafter.
“If browsing for shoes, also recommend spray.”
These first-generation systems used people, rather than predictive algorithms, to make recommendations.
Especially in categories with large selections, such as books, videos, and content, recommender systems using the so-called wisdom of the crowd are more effective. Actual usage data or review data from users has more information than metadata like title, description, and keywords that describe the content. When we are trying to find a restaurant, book, or a movie, we tend not to trust canned descriptions of the product we are looking to buy.
Instead, we ask trusted friends and colleagues what they think. We use the same logic with recommender systems, which can figure out which customers are most similar to an individual user and use behavioral data (usage, reviews, purchases, views, downloads) to recommend other content for that person. This strategy will allow for more relevant recommendations rather than just trying to recommend products based on certain labels or content. In mathematical terms, these user-based recommendations are called collaborative filtering.
Choosing the right customer or segment
The first question to answer is who to make a recommendation to and when. Good times to make recommendations are either during a purchase or after a purchase and at certain times during a customer’s lifecycle, such as when you have not heard from a customer for a while. These recommendations are referred to as upsell, cross-sell, and next-sell recommendations respectively.
Recommendations made at the time of purchase
Upsell and cross-sell recommendations can be made to customers during a purchase, served on a website’s product page, or during checkout.
A basic example of upselling is asking a McDonald’s customer if she wants to supersize her meal, but similar instances can be found in all industries. You could suggest a high-end version or a multipack of the same product, perhaps at a better price. Upsell recommendations are typically tied to a specific product: Each product has other suggested products that can be used as upsells.
Cross-sell recommendations are also made at the time of purchase. Rather than recommending buying a larger or better version of a specific product, cross-sell recommendations are made to suggest other products that are typically bought alongside this specific item.
The recommendation could read: “Customers who bought a printer also tend to buy printer ink…,” and you could offer a modest discount if the customer decides to buy your cross-sell bundle. Like upsell recommendations, cross-sell recommendations also tend to be tied to specific products: Every product has suggested products that can be used as cross-sells.
Upsell and cross-sell recommendations are a great way to increase average order value. Most upsell and cross-sell recommendations are tied to the product rather than to a specific customer.
Recommendations made after a purchase
Next-sell recommendations are typically made after a customer has already made a purchase. This type of recommendation could be included in a thank you page or in the confirmation email.
The best next-sell recommendations are specific for each customer and take into account more customer data than just the most recent transaction. By the time somebody has completed a transaction, you know who this person is and should be able to make a more personalized recommendation. The more you know about a person, the better the recommendation. So if you can analyze all the purchases that a person has made, both online as well as in-store, your recommendations will be more accurate than if you are only looking at online transactions.
Recommendations made during the customer lifecycle
You can try to use recommendations to reengage or reactivate lapsed customers. In this case, first you use predictive analytics to identify a group of customers at risk of leaving. Then you can reengage these customers with a personalized email.
The recommendation can be a product, content, or relevant person. The power of recommendations is that they can be dynamically inserted into a web page or email and create an entirely personalized experience without having to redo the creative for each customer. The web page or email design is the same for every customer. Even the text in the page or email could be the same, telling lapsed customers, “We miss you. Please come back soon. We have these products waiting for you!” while including person-specific recommendations.
5. Predict likelihood to buy
Propensity models, also called likelihood to buy or response models, are what most people think about with predictive analytics. These models help predict the likelihood of a certain type of customer behavior, like whether a customer that is browsing your website is likely to buy something. We’ll examine how marketers can optimize anything from email send frequency to salesperson time when armed with information about likelihood to buy or likelihood to engage.
One online pharmacy company sells many products that customers have to reorder at varying times from 3 months to 12 months. Like most retailers, the pharmacy took a one-size-fits-all marketing approach, offering a set calendar of discounts and promotions to all customers. But not all customers are alike, and many are looking to buy at different times of the year. Using predictive analytics, the brand was able to differentiate discounts across customers, leading to higher sales and retention without increasing costs. Customers were ranked according to their likelihood to buy.
Based on that ranking, the business was able to determine which discounts would obtain the optimal response from each customer and offer minimal discounts through email or mailed postcards to customers who were already deemed likely to buy and offer larger discounts for customers who were less likely to buy. The surgical promotions drove incremental margin from customers who were already motivated to buy and incremental revenue from customers who previously felt no incentive to buy. Thanks to this and other predictive marketing campaigns, quarterly sales increased by 38% from the year before, profit rose by 24%, and customer retention increased by 14%. Plus, the changes allowed the pharmacy to more than double its campaign response rates without increasing the marketing or promotional budget by a single dollar.
Likelihood to buy predictions
To predict which prospects are ready to make their first purchase, a likelihood to buy model evaluates non-transaction customer data, such as how many times a customer clicked on an email or how the customer interacts with your website. These models can also take into account certain demographic data. For example, in consumer marketing, they may compare gender, age, and zip code to other likely buyers. In business marketing, relevant demographics may include industry, job title, and geography.
Here’s how it works: The models compare the pre-purchase behavior of prospective buyers to the pre-purchase behavior of thousands or millions of previous customers who ended up buying, comparing attributes like what emails they opened and what products they spent the most time looking at.
The prospects that behave most like the previous buyers are tagged as “high-likelihood buyers,” and marketers can then alter the way they interact with them to increase the likelihood of closing a sale. Once you’re armed with this data, you can prioritize your investment in each prospective customer.
Likelihood to buy for first-time buyers
For consumer marketers, likelihood to buy predictions allow you to decide how much of a discount you might allocate to a certain customer because people who are already more likely to buy won’t need as aggressive of a discount as customers who are less likely to buy. The models then get better over time, as companies collect more data and automatically test whether predictions actually become reality.
For instance, the large European household appliance manufacturer Arcelik maintains a call center where employees are given a list of customers who are likely to be ready to buy a new washing machine within the next few months. Agents then make calls to these customers with offers such as a year of free detergent with the purchase of a washing machine. The tactic works well for considered purchases, such as refrigerators or cars, and larger-ticket items such as high-end fashion apparel. A high-end shoe brand provides store associates with lists of customers to call too. The store associates have already developed strong relationships with their customers, but they can be even more successful when armed with predictive analytics. Employees can now see which customers are likely to be interested in a certain style when a new season’s shoe comes out based on customers’ past behavior or how similar their purchase habits are to other customers.
Employees can then reach out to customers with that information. A call could go something like this: “Hi Joe, it has been a while since we’ve spoken. I just wanted to let you know that there is a new cross-country running shoe I think you might like. It’s similar to the shoes you bought two years ago but in a new material. I have put a pair aside for you in your size. If you have time, perhaps you could stop by on your way home from work to have a look?” Who would not want to receive a call or an email like that from their personal shopper?
Likelihood to buy for repeat buyers
What good is spending money to acquire new customers if they only buy once and do not return? Therefore, it is not only important to predict likelihood to buy for first time buyers, but it is equally important to predict likelihood to buy for repeat buyers. Your goal is to keep customers coming back time and time again. It is happy and loyal customers who have a large lifetime value, and many customers with a large lifetime value make for large revenues and profits for your company.
Predicting likelihood to buy for repeat buyers is a lot easier than predicting likelihood to buy for first-time buyers because there is a lot more information to go on. The likelihood to buy model for repeat purchases evaluates earlier transactions as well as other interactions similar to the model for prospects. However, the added information derived from the first purchase can significantly improve the accuracy of the likelihood to buy model for repeat purchases as compared to a similar model for prospects. Unlike the first purchase predictions, repeat purchase predictions utilize all interactions of the customer, such as past purchases, returned purchases, and phone calls to customer service.
6. Customer reactivation campaigns
When a customer leaves you, not all is lost. Our data shows that it is, on average, 10 times cheaper to reactivate a lapsed customer than it is to acquire a new one. Reactivation programs for lapsed customers are a low-hanging fruit for marketers looking for new revenue streams.
Reactivation campaigns are for customers who have not purchased anything for an extended period of time. Typically, a customer is considered lapsed or lost after she has not spent money with you for twelve months.
One company we work with noticed it had a large number of customers who once loved the brand but hadn’t engaged with the company for a while. It wanted to focus on customer loyalty and engagement by bringing enthusiastic customers back to the brand. The company used its knowledge of the type of products its customers enjoy to send smart product recommendations. These campaigns resulted in an eightfold increase in monthly revenue.
Reactivating customers builds on the investments you have already made and avoids the costs of trying to get new customers. These original customers are already aware of your brand and are more receptive so reengaging them can lead to gaining significant incremental revenue.
In many measurements we made, most reactivated customers behave like a new customer; that is, they almost restart their lifecycle. This also means that the early periods after reactivation are when customers are most vulnerable to lapse again and require special attention.
Reactivation campaigns in four steps
Where do you start with reactivation? First, determine which customers you want to reactivate. Then determine the most receptive candidates, customize your message to this group, and reengage these customers using different channels.
1. Determine which customers you want to reactivate
Not all past customers may be worth bringing back. Marketers need to carefully determine which customers were profitable, interested in products they want to sell/grow, and other strategic factors.
2. Determine the most receptive candidates
You may only want to approach past clients who are most likely or ready to respond — especially if your reactivation campaign is expensive, such as in the case of direct mail or targeted display advertising. Even if you are using email, you probably don’t want to send marketing messages to customers who are not ready — otherwise you risk seeming overenthusiastic and driving customers away.
3. Reengage customers using different channels
The shopping journey spans across many distribution channels. There is no reason your marketing message should not be omnichannel as well. After customizing a message using your customer’s past data, try to reach customers at as many points as possible. A greater number of contacts usually correlate to a higher response rate. So don’t forget to send that email, postcard, or app notification with personalized offers.
Customize marketing message using past data
Now that you know which lapsed customers are ready to respond, take a look at their purchase history. What have they responded to in the past? What type of products do they buy? What kind of brands do they like? Craft your messages according to their tastes and needs. By looking at past trends, you may be able to figure out why the customer lapsed and use this as an opportunity to address this reason. Perhaps the customer may give you a second chance.
7. The predictive analytics process
We’ve looked at examples of predictive marketing use cases and ways marketers can use predictive analytics to understand customer behavior. Now that we’ve explored the insights these techniques can generate, let’s walk through the different steps a data scientist or analytics software goes through to make accurate predictions or recommendations.
Figure 1 gives an overview about what is happening under the hood, either of out-of-the-box predictive analytics software or the steps your internal data scientists will have to go through if you are building your own predictive analytics models.
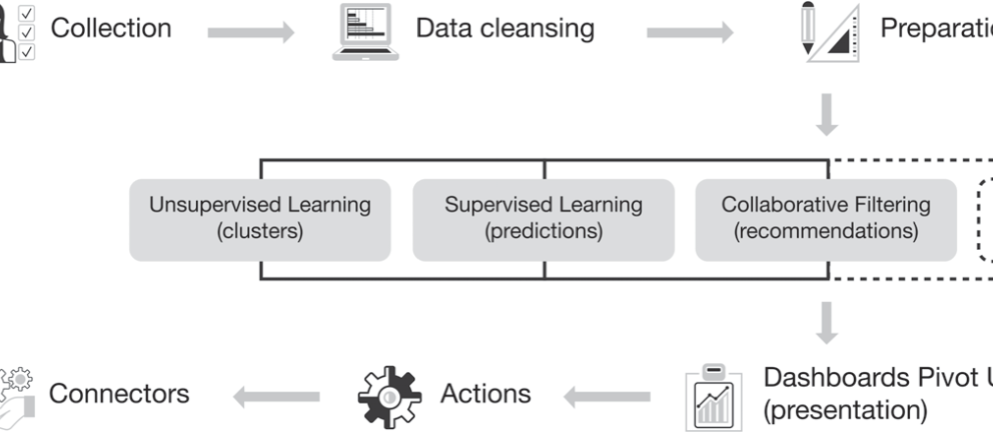
Data collection, cleansing, and preparation
Data cleansing and preparation is the most important and most ignored stage in predictive analytics. In some cases, the data might be missing or incorrect as collected. Data cleansing is used to correct things like names and addresses to make sure the computer will know that a customer lives in California when her state is listed as CA.
You can learn more about the processes used to unify and standardize individual customer profiles in our e-book Identity Resolution 101. |
However, even after building 360-degree customer profiles, there still is significant work to be done getting your data ready for analysis. Not all data collected is immediately usable, and the results could be skewed by missing data or outliers, data measurements that are either too low, too high, or do not fit the underlying data-generating system.
Outlier detection
Outlier detection often makes a big difference in the accuracy of predictive models. For example, if a customer at an electronics retailer came in and bought 50 televisions for $50,000 when the average customer at the retailer spends $500, this high-spender will skew the average order value metric. In electronics retailing, these types of outliers, where there are few users making large purchases, are indeed quite common.
People making such large purchases could be middlemen who are buying items like televisions to take out of the country and resell. These are not normal consumer customers but rather gray market resellers. If this situation wasn’t recognized and corrected, the retailer would think these are great VIP customers. Not recognizing this creates two problems: distorting the definition of VIP customers so the true VIP customers would be left out and masking an opportunity to market to this group of resellers in a more profitable way.
To correct for the outlier, your data analyst or predictive marketing software will need to detect and either remove the outlier or replace it with a number at the high end of the distribution (e.g., The lowest spend of the top 10 percent of customers is $2,400, so replace the $50,000 with $2,400).
This replacement is only done for modeling purposes. Alternatively, you can treat these customers as a separate group altogether and create specialty programs for this one segment.
In another example, one retailer was measuring foot traffic at each store but would miss out on data for certain days whenever the measuring device would get knocked off by the cleaning crew. To correct for the missing data, the retailer applied an imputation based on the three-week average for the same days in the week as the missing days.
Imputation is the art and science of replacing wrong or missing information. Depending on the specific data elements, there are various techniques for this:
- Replace with static or temporal averages.
- Model the data based on other variables available. For example, you can model a vitamin store customer’s age based on whether she buys vitamins geared toward women above age 50.
- Random selection from the underlying distribution. For example, if the foot traffic data is missing and this data usually follows a bell curve, then randomly generate a number from the underlying distribution.
Imputation is a great way to make up for missing data until the problem is corrected at the source. Another example of imputation is asking customers for their birthdays. This is a great piece of information for modeling and action purposes but not all customers want to provide this information. In such cases, the predictive model would either discard the birthday as an input or discard customers with no birthday.
Feature generation and extraction
Once your data scientist or predictive marketing software has cleansed the data for missing information and outliers, there are two other factors to consider: (1) The data may be too large to use as is, or (2) data in its current representation may not be suitable for the models.
Feature generation and extraction turns data into information that the models can digest and discards unnecessary or redundant information.
Think of feature generation and extraction as separating the signal from the noise. Feature extraction deals with removing unnecessary information by either throwing it away or transforming it to eliminate the noise. There are quite a few mathematical methods to use, but the short explanation is to utilize algorithms to be able to extract the maximum amount of information from the data, regardless of what you will use it for later on. This optimal extraction leads to less noisy data; hence, increasing the accuracy of predictive analytics.
Classifier and system design
The next stage in the process used by data scientists or predictive marketing software is choosing, architecting, and fine-tuning the correct algorithm. In machine learning, there are two important concepts that need to be understood.
One is the “no free lunch” theorem, which states that there is no inherently better algorithm for all problems out there. This is important to understand, so the data scientist chooses the right algorithm for the right problem and doesn’t use the same algorithm for every problem.
The other concept is called the “bias-variance dilemma,” which states that if you go deep in developing an approach and algorithm to solve a specific problem, then the system that is biased toward this specific problem gets worse at solving other problems.
The lesson learned here is to understand that no algorithm is inherently better than the other. If you develop your own algorithms, it means you probably have to develop multiple algorithms for multiple situations. If you buy off-the-shelf predictive marketing software, you want to make sure to choose a vendor that focuses on your specific vertical and business problems and/or to choose a vendor that has self-learning algorithms that can adjust to your specific situation automatically.
The last mile problem of predictive analytics
Most data scientists don’t worry how marketers will use their predictions. Frankly, most data scientists don’t know enough about marketing and marketing systems to embed predictions into the daily routine of marketers.
An email marketer at a large national department store once told us: “Brides register with us on our website and leave a lot of personal information. That information is in our customer data warehouse somewhere, and we probably even analyze it. However, as an email-marketing manager, I am unable to run a simple campaign that takes into account some of the preferences or dates that the bride has shared with us.”
We call this the last mile problem of predictive analytics.
Especially in organizations with in-house data scientists, the outcomes from predictive models are often not easily digestible or usable by marketers. It is often very difficult for marketers to put predictive analytics into action — to connect the dots from analytics to the daily campaign management of email, web, social, mobile, direct mail, store marketing, and customer interactions in the call center.
For customer predictions to be profitable, predictions need to be put in the hands of all the customer-facing personnel in your organization. If you can’t surface recommendations to the personnel in your call center, the upsell might never happen. If you can’t use likelihood to buy segments to decide whether to send an abandoned cart holder a discount or a reminder, you are leaving a lot of profit on the table.
8. What is customer lifetime value?
Customer lifetime value is a term that describes how much revenue or profit you can expect from customers over their lifetime doing business with you. There are a couple of different ways to calculate and use lifetime value, depending on the marketing problem at hand.
Historical lifetime value
Historical lifetime value (LTV) is defined as the actual profits — gross margin minus direct costs — from customers over their lifetime so far, adjusted by subtracting the acquisition cost of those customers. Note that historical lifetime value only takes into account past purchases, not future purchases. The only time to use historical lifetime rather than predicted customer value is when you are trying to detect if the customer value of a specific customer or customer segment is trending up or down.
A customer might have spent $500 two years ago but only $200 in the last year. It is this change in lifetime value that signals underlying trends, risks, and opportunities. If a customer’s historical lifetime value is trending down, this is called value migration, and this can be an early warning signal of customers unsubscribing from your service or planning to stop buying from your website. Detecting value migration allows you to catch customers before they walk out the door, and it is too late to win them back. Identifying a change in historical lifetime value allows you to implement a reactivation or proactive churn campaign to turn the tide.
Beyond value migration, customers may be changing their spending habits in other important ways. A certain customer may have made only one big purchase last year, but this year they are making smaller purchases more often. While the customer lifetime value of this person has not changed, your marketing approach and goals for the person should change. To accurately calculate historical lifetime value, you need to be able to link all purchases made by the same person — even if that person used slightly different emails, names, or addresses.
We recommend that you take the cost to service a customer into account whenever you can in order to calculate historical lifetime value. This includes returns and discounts, as well as product cost. On average, 9% of all retail sales in the United States are returned by consumers, so ignoring returns would skew the results. Some practitioners calculate LTV without the acquisition cost. If LTV is being utilized to make acquisition decisions, acquisition cost should be taken into account. However, if it is for existing customers, acquisition cost is a sunk cost and should not be used.
Predicted customer value
Predicted customer value is the projected value, revenues, and costs adjusted for the time-value of money of a customer looking forward several years. Your average retention rate will tell you how many years in the future on average you will retain a customer and how many years of future revenues to take into account. We typically look one to three years ahead when calculating predicted customer value.
Predicted customer value is very useful, especially when deciding how much money to invest in acquiring or retaining a specific customer. If you were to only look at historical lifetime value, you would significantly underestimate the potential of a customer and likely underinvest in the acquisition or retention of certain customers. It can also be used to identify high-value customers very early in the lifecycle. After her first purchase, a future high-value customer looks just like everybody else. If you could recognize the high-potential customer early in the lifecycle, you could start differentiated treatment right then and there and increase the odds this high-value customer will stick with you.
One person might have just bought this expensive jacket, but he might have only been a customer for two months, but another customer might have been a customer for five years and bought the same jacket. If you were to look at historical lifetime value, you might draw the conclusion that one customer is more valuable than the other.
However, these two might very well become equal value customers and should probably be treated in much the same way. If you look at historical lifetime value, you look too much at old customers and will miss the opportunity to acquire or retain more recent, high-potential customers. With predictive analytics, you can estimate the future value of a customer by comparing a customer to the thousands or millions of others that have come before them. You can predict future lifetime value by finding customers that look just like them. From the example we used earlier, buying a certain type of jacket may very well be an early indicator of a known pattern of behavior for a high-value customer. Even if predicted customer value is not accurate in absolute dollar terms, the rank order it provides gives the marketer focus on the right segment and trends.
Here are some examples of factors that can signal future lifetime value. Predictive marketing software typically looks at hundreds of factors like these but will only use those that actually correlate with future lifetime value in your particular company or situation:
- Recency of engagement: The recency of purchases, web visits, reviews, and email clicks may all be important predictors of future purchases and thus future customer value.
- Size of the first order: Customers who make a large first order are more likely to end up being valuable shoppers.
- Discount on the first order: Customers who buy full price are more likely to become valuable over their lifetime.
- Multiple types of products in the first order: Buying from different categories, such as shoes and electronics, in your first order is a signal of future customer value.
- Time between orders: Most valuable shoppers make frequent purchases, and thus a shopper who places a second order quickly is more likely to become a high-value customer.
- Time spent on a website: The more time prospects or customers spend on your website, the higher their likelihood to buy and the higher their predicted customer value.
- Social and email engagement: Customer engagement of any kind, including email opens and clicks or social engagement, are great predictors for likelihood to buy and predicted customer value. Often, it is not the amount of engagement that matters but the consistency or frequency of engagement. Spending a little time every day is a more reliable indicator than spending hours sporadically.
- Acquisition source: It turns out that certain channels drive higher value customers than others. The customer who came from a fashion blog may have a higher predictive value than the customer acquired through a banner ad.
- Geography: Customers in certain zip codes have a greater predicted customer value than others. Rural populations tend to be more stable, move less frequently, and therefore have more loyal purchase behavior. Zip codes can sometimes predict what type of products people buy. For example, zip codes with many apartment buildings have a low predicted customer value for certain products, such as lawn mowers.
- Seasonality: Retail customers who are acquired during the holidays tend to be about 14% less valuable than those acquired during other times of the year.
- Personal referrals: People who came to your brand through a personal referral tend to be more loyal than those who buy because of an advertisement.
Predictions about lifetime value are not destiny. Marketers can do much to change the course of history here.
Take, for example, the fact that shoppers acquired during the holidays tend to be less valuable and less loyal than shoppers acquired at other times during the year. One skincare company decided to focus its retention efforts on this holiday cohort specifically. It set up an email marketing campaign to increase brand loyalty among new Cyber Monday customers, sending regular reminders for refills and recommending other products of interest. They were able to reverse the trend, and lifetime value of these new holiday customers is now 5% higher than the company average.
By focusing on specific outreach to underserved customer segments, the company was able to offer personalized promotions that ultimately drove greater brand loyalty. The important lesson is that, once customers are acquired, the best strategy is to focus on engaging them to grow and retain them, ignoring the cost of acquisition.
Upside lifetime value
Upside lifetime value, which is also called size of wallet, calculates how much more money a customer still has to spend with you. This is money that the customer is already spending at your competition to buy the products you offer. Algorithms can figure out size of wallet by comparing a customer to other like-minded customers.
It is important for marketers to focus on what size the wallet is, because it is always easier to grow a relationship with an existing customer than to acquire a new one. Unfortunately, most marketers have been taught to focus more on new customer acquisition than on engaging and retaining existing customers.
Especially if the customers have high upside potential, marketers should focus on how to deepen their relationship by introducing them to new products or serving them in a differentiated way. Very few companies calculate and utilize the upside or share of wallet potential of a customer, yet it can be a very powerful way to identify customers to focus on.
The critical difference between future lifetime value and share of wallet is often in the types of products that are factored into the analysis. For future lifetime value, you tend to look at just those products that a customer is already buying from you.
For example, I may be a hockey player and I may be buying my hockey tape from a specific outlet every couple of months. Based on this, the company can project that if they retain me I will buy a lot more hockey tape in the future and perhaps have a predicted lifetime value of $300. However, because I am buying hockey tape, I am likely in the market for skate sharpening, hockey sticks, and occasional gear upgrades. I am clearly buying those things elsewhere right now. If I were to buy all of my gear at the same place I buy my tape, my future lifetime value is probably well over $1,000.