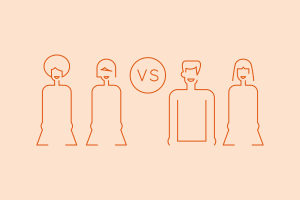
Collection
What’s the Difference Between Segmentation and Clustering?
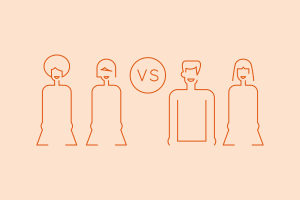
Collection :
Marketers have been using segmentation and clustering for some time now. Both are important facets of observing and predicting consumer behavior, but there’s still confusion about how they differ and how they affect your marketing.
We’ll dive in shortly, but let’s talk about both generally first: Clustering and segmentation both involve grouping people based on similarities. In the land of digital experience, they’re the dynamic duo of predictive marketing. The main difference between the two is that clustering is generally driven by machine learning, and segmentation is human-driven. This difference has caused more than a few folks to be clustering-averse and to cling to their own customer knowledge.
That reluctance touches upon the familiar battle of man versus machine. But instead of seeing segmentation as the human version of data grouping versus the machine version of that same act, these tactics can be used together. The dichotomy is unnecessary. In fact, as you’ll see, they can be used quite powerfully together.
Both tactics help you understand customers and prospects better, and the more you know about how people interact with your organization, the better you’re able to give them what they want. That’s money in the bank, good customer experience, and smart business.
What is clustering?
Clustering uses machine learning (ML) algorithms to identify similarities in customer data. Simply put, the algorithms review your customer data, catch similarities humans might’ve missed, and put customers in clusters based on patterns in their behavior.
Some examples include finding shoppers who tend to buy a full outfit as visually displayed rather than self-coordinating and buying apparel pieces separately. Another example is vitamin shoppers who like to stock up on promotional offers versus those who restock on a regular cadence. Those that “stock-up” on vitamins, make-up, or other items may not be back to shop for an extended period of time. It may take some creative messaging and offers to get them to purchase outside of their normal cycle.
When to use clustering
Machine learning is a computerized superpower at your disposal. Therefore, it’s best to use clustering when you want to parse through datasets that are so large even a team of human data scientists would take too much time. Clustering is also helpful because, as much as I hate to say it, humans are fallible and a machine might see what we miss in a dataset. This allows you to potentially target clusters across your entire customer database, allowing you to personalize at a higher level, and much faster than we humans can.
How to apply clustering to your marketing
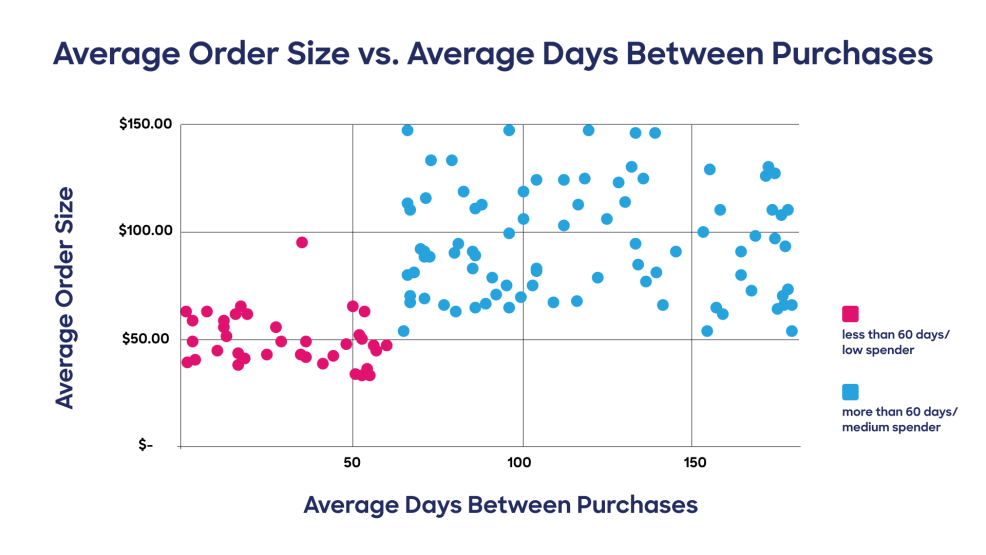
Here's a simple example of the machine learning clustering algorithm finding similar groups of data in a large dataset. In this example, the more frequently a customer buys, the less they spend. Contrarily, the longer the buying cycle, the higher the spend. This is what the data would look like when comparing Days Between Purchases vs. Average Order Size. In the example of “stock-up” buyers, this is what we might see (less frequent purchases but for a lot higher dollar value). Keep in mind that machine learning clustering algorithms are doing this comparison across dozens and, in some cases, hundreds of data points on a multivariate level to identify clusters of customers with similar behaviors. It’s just not possible for humans to perform a task at this scale.
The main point is that machine learning powers clustering and that the algorithms will find similarities; marketers don’t do any work, per se. Instead, they decide what steps to take after ML has done the initial work.
For instance, in the example above, a cluster of buyers taking over 60 days to place a second order were identified. Using that data, marketers can develop targeted campaigns that pinpoint that cluster. Because if there’s such a similarity in buying patterns for “stock-up” buyers in that group, perhaps they share patterned behaviors for other products. In a case like this, a marketer might consider sending this cluster a discount code for vitamins or supplements outside what those buyers are normally purchasing.
What is segmentation?
When a marketer chooses criteria to pull certain groups from a large body of data, that’s segmentation. Put another way, it’s when you look at your customer data and pick out specific criteria to target a group.
When to use segmentation
This is where human beings use their heads and, weirdly enough, data informed hearts to key in on certain groups and drill down as specifically as they want. Where clustering informs, segmentation empowers and allows the marketer to select and purposefully target specific groups for personalized messaging.
How to apply segmentation to your marketing
With segmentation, you (a human being) choose your target.
If I’m selling vitamins, I may want to target customers with messaging that speaks to the types of products they have purchased in the past. I may want to segment customers who have purchased fish oil, folic acid, and magnesium in the past with a heart healthy message. I may want to segment that group even further by selecting gender, age, or lifetime spend. Deliberately identifying and grouping customers who have similar buying habits and a proclivity toward certain categories is an example of segmentation.
Where segmentation can be error prone is in human assumption. I assume that this segment is purchasing these items because they are interested in heart health and, because of their ages or gender, may need it more. Clustering on the other hand, may find patterns in buying behavior unrelated to the attributes we are considering. Sometimes our biases can mislead us; they push us to select a segment of people we think we should target.
Clustering doesn’t have preconfigured biases; it just crawls data for similarities.
But identifying this segment is still important because I can use this in conjunction with clustering to expand my audience. By customizing how I market to this segment, I increase their likelihood to buy and, by extension, the conversion rate.
Machine learning in a cluster analysis helps by constantly analyzing behavior and rescoring customers. With this daily iteration, cluster analysis looks for behavioral changes including web behaviors and email engagement behaviors that would be impossible for a human to monitor on a daily basis. Together, segmentation and clustering are powerful allies.
Customer data platforms: The technology you need to segment and cluster
With the rise of big data, marketers now have hundreds of characteristics they can study: brand preference, discount preference, time spent on site, browsing behavior, and so on. Some customer characteristics have no correlation to buying behavior; other characteristics correlate to buying behavior and to each other in different ways.
But it's not feasible for a person (even a team) to go through hundreds of data types to find relationships between each. Without a dedicated data science team or other technology that enables ML, this would be an impossible lift. Machine learning models in a customer data platform (more on CDPs in a minute) can go deeper and parse through thousands of data sets to predict buyer likelihood through cluster models.
But tracking and strategizing based on human behavior across digital properties, as well as identifying duplicates and resolving or combining data points, can be a lot.
Identity resolution technology offers a solution. For instance, through identity resolution, you can figure out that the same person uses two different email addresses. Although it’s a simple example, you can imagine how multiplying the issue by thousands could lead to data chaos.
Fortunately, we have technology that solves these issues. I mentioned them earlier, but customer data platforms (CDPs) are an ideal place to house and consolidate all your customer data so you can cluster and segment accordingly. A CDP ensures you don’t have all sorts of customer data spread between disparate systems. Instead, it acts as a single source of truth with 360° customer profiles — aka data that gives the most accurate snapshot of behaviors around which you can build personalized experiences. CDPs that incorporate identity resolution are especially valuable.
And CDPs that feature machine learning make personalization efforts dynamic. Because human beings aren’t consistent creatures, their behavioral data may be puzzling. For example, a CDP will decipher the difference between a user browsing and actually buying something versus a user “window shopping” and filling a cart with stuff they’re never going to buy. Knowing this, marketers can recommend similar items they could purchase versus sending a gentle reminder that they’ve left their cart full or offering a coupon for an order over a certain dollar amount.
Thus, customer data profiles, clusters, and segments are constantly being examined to study changes and inform strategic decisions accordingly. Machine learning constantly performs tedious analyses to yield clusters, while you choose the parameters of your segments. In both cases, darling human, you decide the marketing approach you’ll use based on the insights that each tactic produces.
Next steps to take
Let’s recap.
- Segmentation: Manually pulling certain groups that meet chosen criteria from a large body of data
- Clustering: Using machine learning to identify similarities in customer data
Both complement each other, and the main difference is that segmentation involves human-defined groupings whereas clustering involves ML-powered groupings.
The amount of customer data that modern businesses handle is staggering. Successfully weaving clustering and segmentation into your marketing tactics depends on how you organize your data, which is where a customer data platform can be a huge help.
For more ways marketers can use a customer data platform to organize and activate their data, download our free e-book, Working With Customer Data: From Collection to Activation.
Or, if you want to see it in action, we’ll be happy to show you how it works in real life. Schedule a demo here.
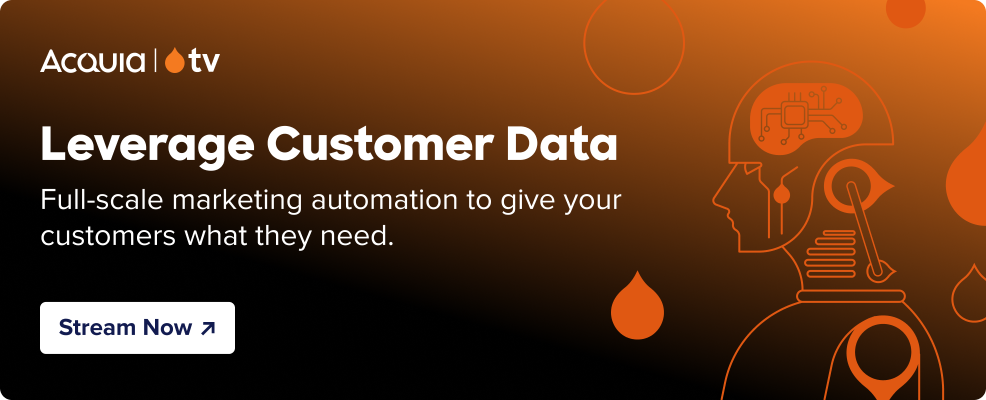