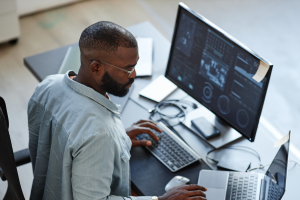
Machine Learning Models to Consider for Your CDP
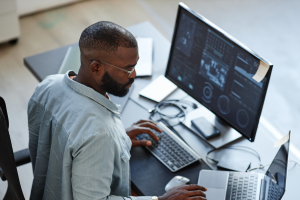
A self-driving car that adapts to road conditions in real time. The Facebook prompt of your mom’s name when you hover over her photo. The auto-fill that correctly guesses what you would have typed into Google’s search bar.
All examples of machine learning (ML) in our everyday lives. All examples of ML’s power.
By processing large data sets more quickly than any human ever could, machine learning, a subset of artificial intelligence, can accurately predict our behaviors and tendencies.
Imagine that power embedded in a customer data platform (CDP), software that collects and unifies data across channels and systems to create a single source of truth for customer data. With a CDP, organizations can more finely tune marketing campaigns, derive business insights that guide operations, and craft initiatives that lower churn.
No wonder more and more organizations are looking to add a CDP to their technology stack. Indeed, according to the CDP Institute, industry revenue is expected to reach $2.5 billion in 2024.
This figure points to the increasingly crowded market for CDPs, but not all vendors’ machine learning capabilities are the same. Many will claim to incorporate machine learning in their product; many will fall short. To help organizations separate the signal from the noise, we partnered with the CDP Institute to provide advice for marketing decision-makers. The resulting white paper reviews organizational use cases that favor the technology, different kinds of ML models, and what to look for when evaluating the claims of would-be CDP vendors.
Use cases: How a machine learning-enabled CDP helps organizations
Let’s dive into use cases of ML-powered CDPs to get a better sense of the many ways that this subset of AI is changing how organizations approach customer data.
Acquiring new customers
Growing the number of new customers is a must for any business, and a CDP with ML capabilities is a critical tool for reaching that goal. For instance, it can help with media optimization and choosing the best offers that attract certain segments. Importantly, it can also estimate the long-term value of particular customers, facilitating improved targeting.
Increasing customer lifetime value
ML-powered CDPs not only help organizations expand their customer base, they can also grow their lifetime value after acquisition. Such CDPs can, for example, determine the best path forward when a customer is active in multiple campaigns. Or, if there are product categories that a customer hasn’t yet bought into, an ML-enabled CDP can evaluate those that have the highest cross-category purchase likelihood. And, using channel-optimization models that take into account a customer’s channel preferences and potential response rates, ML-backed CDPs can offer the most effective messaging channel for each customer.
Improving customer experiences
Providing excellent customer service on- and offline remains a top priority for competitive organizations, and a CDP can help in both arenas. For example, a CDP can use ML models to predict the level of support individual customers may need, as well as suggest the best course of action to customer service reps and chatbots. Optimizing offline service operations may include recommending what inventory would be best added to field service vehicles or the best routes for field engineers and delivery drivers.
Maximizing internal operations
CDPs can also assist organizations with activities that don’t involve customer interactions. For instance, they can lean on ML models that help businesses forecast demand, thus improving how inventory is managed, as well as analyze the value of product features or why some products fail. So, beyond customer management, ML-powered CDPs can support supply chain and product design teams.
Complying with data privacy regulations
Perhaps one of the biggest benefits of ML-supported CDPs is their ability to ensure compliance with data privacy standards, which are on the rise. CDPs achieve compliance via ML models that use master training data sets organized by permissibility. For example, a training data set can exclude privacy-sensitive data altogether, but if some customers have consented to the use of their data, then the data set may include that information. Some ML systems can also detect sensitive items in the data and ask users whether or not they should be included in the model.
Unifying data through identity resolution
Unified data may be the key output of CDPs. ML applications produce these so-called golden customer records via identity resolution that combines data from various sources. Even when there’s no shared, persistent customer ID, some CDPs can merge the data and include exact, deterministic, or probabilistic matches. (Be sure you know which match types you need so that you choose a CDP that can deliver them.)
Types of machine learning models
The small handful of use cases mentioned above hopefully offers a glimpse into the wide range of applications to which CDPs may be put. Just as far ranging are the kinds of ML models needed to produce the results organizations crave. Let’s take a look at three primary categories of machine learning models and some examples within each.
Predictions
One of the most familiar purposes of predictive models is to anticipate future behavior. By observing behaviors over time, brands can more accurately judge customer lifetime value and create more relevant experiences. Here are just a few examples of the types of predictive ML models a CDP can create:
- Likelihood to engage: ML can predict the odds a customer will open an email or subscribe to a newsletter, informing which segments to send certain offers to.
- Likelihood to buy: This model predicts if a customer is at the point in their journey to make a purchase. This information is valuable for knowing which users to nurture more and which to offer time-sensitive discounts.
- Likelihood to churn: This model identifies at-risk customers so an organization can be prepared for a loss of income or focus on a win-back campaign.
- Likelihood to pay full price: This model predicts the degree to which a customer is likely to purchase a product without a discount, so brands can increase revenue by getting more full-price conversions and reduce the time and resources spent sending irrelevant offers to this audience.
- Predictive lifetime value (PLV): This model predicts the revenue or margin of a customer over the course of the next 12 months by looking at their actions across different channels from in-store purchases to email engagement to web-based behaviors like session duration and cart abandonment. By identifying the most engaged, highest value customers, an organization can focus their efforts on nurturing this existing core audience.
Personas
The persona category comprises a series of clustering models. Clustering is an unsupervised learning technique where the machine learning algorithms create customer segmentations based on many different variables. Unlike marketer-made segments, machine learning models can take into account many more customer dimensions. A few types of useful clustering models for marketing include:
- Product Clusters: Knowing which types of products certain segments regularly purchase helps improve targeted campaign efforts.
- Behavioral Clusters: These clusters reveal things like preferred channel, average spend and average time spent browsing vs. buying so marketers can better anticipate how, when, and where to engage.
- Seasonal Clusters: Many retail brands use seasonal segmentation and data to detect patterns of when customer demand is high for certain products and inform when to start “summer sales” or begin heavily discounting season-specific items like overcoats and snow boots.
1:1 personalization
Today’s customers are constantly inundated with new marketing messages from brands, and the influx of new digital touchpoints means that the stakes to create memorable connections and earn customer loyalty are higher than ever. Personalization empowers marketers to create a holistic marketing strategy that covers the who, what, where, when, and why of customer experience. Here are some examples of how personalization models can be used to reach the right audience with the right content on the right channel at the right time:
- Next best product recommendations: the “what” a customer wants to buy
- Send time optimization for email campaigns: the “when” customers want to receive messages
- Next best channel: the “where” customers prefer to interact with brands
Some well-known examples of this are Spotify’s personalized “Daylists” playlists or Amazon’s “other products you may like” feature. By tailoring an experience to each customer’s preferences, marketers are able to boost upsells and cross-sells and keep people within their brand ecosystem longer. And as a bonus, the more actions the customer performs on your site or products they purchase, the more customer data is given to the algorithm to continue personalizing their next best experience.
Requirements to consider
Reviewing which ML-powered CDP is best for your organization involves weighing not just the use cases it supports or the kinds of models it includes. Other considerations include:
- Explainability. Much as we’d like to think that we control AI, some of its outputs exceed human comprehension. To account for this, ML systems offer a range of reporting. Some will identify the most important data elements and explain why particular records were scored a certain way, while other systems will report on a model’s performance over time. The types of reports organizations require will depend on the expected user base. A non-technical user will benefit from reports that a data scientist would find less useful, for example.
- Scale. The size of an organization’s customer base, the amount of data it generates, and the sources from which the data is collected are variables that influence a CDP’s performance requirements. Does your organization enjoy a customer base of millions (and the billions of data points they represent)? Or does your organization have a more modest pool of data sources? Keep scale in mind as you evaluate both what will go into your CDP and what you expect out of it.
- Automation. Do you need a CDP that automates the creation of ML models, or do you need it to automate other ML-related tasks, such as exploring training sets or data preparation? Parse which automation tasks require machine learning and which may be handled by other components of the CDP or other tools included in your marketing technology (martech) stack.
Where to go from here
The ways in which machine learning can bolster CDPs are manifold, and we’ve only scratched the surface here. Plus, the field of machine learning continues to evolve, so the outcomes and models described above only showcase what’s possible today. An in-house data specialist or an enterprising vendor may have requests or planned features that push the discipline’s boundaries, so don’t be shy about asking for the seemingly pie-in-the-sky. At the very least, it will give you an idea of how well-versed a vendor is about the current R&D environment for ML.
In the meantime, we partnered with the CDP Institute to create a comprehensive guide to machine learning and CDPs. Download the free white paper now to enhance your understanding of how ML is shaping the possibilities for CDPs and organizations today!
This blog was originally published in 2023 and has been updated to remain current.