How Artificial Intelligence and Machine Learning Facilitate Empathetic Marketing at Scale
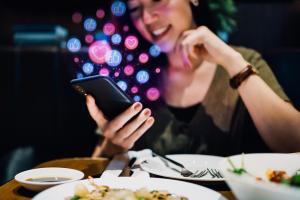
Plenty of organizations want to be customer-centric. Focusing on the customer at every touchpoint helps brands build better relationships, which can raise sales and revenue. It can also cut down on operational costs because satisfied customers require fewer resources — not as many customer service reps, less time processing returns, less inventory, and so on.
Being truly customer-centric goes hand in hand with empathetic marketing, which is marketing through the eyes of the customer. It’s when organizations craft the customer experience (CX) or journey with their customers’ perspectives in mind. That can only happen with the right tools and data.
Sounds counterintuitive, doesn’t it — using software and large data sets to facilitate human connection? But when technologies like customer data platforms (CDPs) are powered by machine learning (ML), a subset of artificial intelligence (AI), the results can generate trust between consumers and companies. With a tool like a CDP, brands can better understand the context and intention behind each customer interaction to design a personalized, empathetic customer journey that responds to a specific individual’s wants and motivations — personalization at its very best. Far from solely transactional, the CX hits at a deeper level.
Let’s explore how CDPs and machine learning achieve that result.
Why ML-powered CDPs are next level
Across industries like retail, healthcare, financial services, and others, CDPs are becoming de rigeur. By unifying data from multiple sources to produce clean customer records via identity resolution, CDPs provide accurate representations of customer behavior, particularly when the tool incorporates ML.
Why is that? Here at Acquia, we define machine learning as a specific set of techniques that enables technology to learn from data and make intelligent predictions. So, when ML is baked into a product like Acquia CDP, the tool takes data points from numerous sources to produce business intelligence (BI) that guides not just marketing departments but other teams throughout an organization. While a human could do the same thing, it would take much longer to manually sift through the mountains of data that organizations collect today and to derive insights based on patterns.
Let’s look at a few examples of what’s possible to get a better handle on just how powerful CDPs can be.
Use cases for ML-powered CDPs
CDPs don’t look solely at current data to arrive at BI insights. They create unified customer profiles spanning multiple touchpoints and past interactions with your brand, such as whether a buyer purchased at a brick-and-mortar location or left a product review. Such data tells you a customer’s history; machine learning uses predictive analytics to anticipate what they might do or want next, not just a week or month from now but in real time too. A brand’s ability to do just that is empathetic marketing to a tee, like when product recommendations align with a customer’s tastes and preferences. Other examples include:
- Your business may be planning widespread store closures as it moves to an e-commerce model. A CDP can ID those customers who shop primarily or exclusively in store, so your marketing team can offer free shipping to soften the transition from their preferred purchasing channel.
- Or let’s say your organization is in healthcare. A CDP can ID patients by their condition and recommend appropriate content, such as clinical trials or treatment options.
- In retail, there may be customers who repeatedly view an item but don’t purchase it. Machine learning can log this behavior and alert such customers via SMS when the price drops. Their response allows organizations to gauge who may be more price-sensitive and send coupons in the future.
- Perhaps business is booming, and your rev ops and business development teams are eyeing expansion into new cities or markets. A CDP can help guide decisions about which locations may best support this initiative by showing existing stores that may be overwhelmed by traffic so could use another brick-and-mortar shop to handle overflow. Or the data may reveal steady overseas sales in Asia. Maybe it’s time to open a branch in Hong Kong.
- Maybe you’re a software vendor for small businesses and freelancers. Based on data from your call or customer service centers, a CDP can help ID consistent pain points, thus guiding product development and perhaps lowering churn. For instance, you could send an email that acknowledges their repeated concerns, shares the product roadmap that addresses those pain points, and offers a discount if they renew their subscription.
So, as you can see, the use cases for CDPs are plentiful and apply to various teams throughout an organization.
What’s next: Empathetic marketing through CDPs
The results that ML-powered CDPs can achieve are remarkable. Machine learning models empower organizations to be more proactive by aggregating and generating insights and recommendations from customer data, helping businesses nurture relationships that better recognize a customer’s interests and preferences. The end result? Customers who feel a brand “gets” them.
That’s the golden objective in empathetic marketing, and a ML-powered CDP best supports it. Be aware, though, that the CDP market is vast, and not all CDPs are created equal or even proper CDPs. Find one that’s RealCDP-certified, like Acquia CDP, which you can learn more about here.