
The Definitive Guide to Predictive Analytics Models for Marketing

Customer data is everywhere. With every customer interaction, marketers collect enormous quantities of information that can inform strategic decisions and shape future behavior. The process of evaluating all of your available data to drive decision making and predict future business outcomes is known as predictive analytics. Rather than just reporting the data, predictive analytics help guide future planning and more confidently anticipate long-term business outcomes.
Predictive analysis also helps marketing teams invest their resources wisely and set KPIs that align with total business value. There are three types of predictive models marketers should know about:
- Clustering models (segments)
- Propensity models (predictions)
- Collaborative filtering (recommendations)
Let’s review each below, as well as 14 examples.
Clustering models
Clustering is the predictive analytics term for customer segmentation. With clustering, algorithms, not marketers, create customer segments. Think of clustering as auto-segmentation. Algorithms can segment customers based on many more variables than a human being ever could. It’s not unusual for two clusters to be different on 30 customer dimensions or more. We call these dimensions the cluster DNA.
The most used clustering algorithms are behavioral clustering and product-based clustering.
Predictive model 1: Behavioral clustering
Behavioral clustering tells you how people behave while purchasing. Do they use your website or call center? Are they discount addicts? Do they consume specific content? How frequently do they buy? How much do they spend? How much time will go by before they engage with you again? This algorithm helps set the right tone while contacting the customer. For instance, customers that buy frequently but with lower priced orders might react well to offers like “Earn double rewards points when you spend $100 or more.”
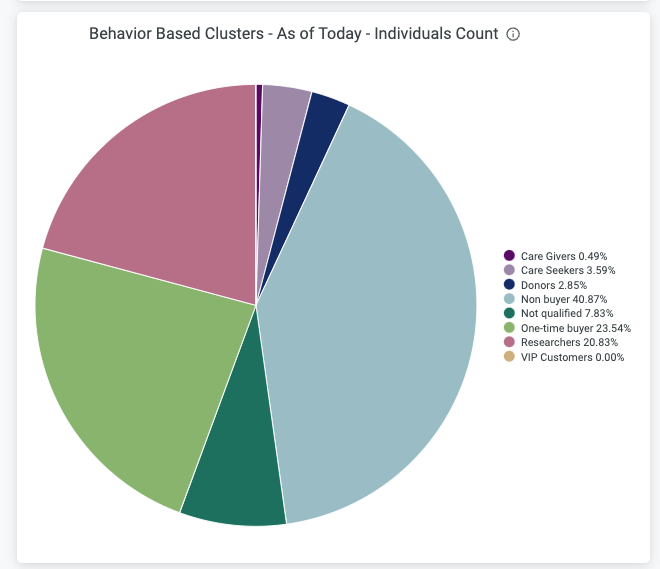
Predictive model 2: Product-based clustering
Product- or content-based clustering — also called category-based clustering — algorithms discover what different groupings of products people buy from. See the example below of a category- or product-based segment or cluster. You can see that people in one customer segment ONLY buy meat products, whereas those in another customer segment buy different types of snacks. This level of clustering can also be applied to content, where you can see that people in one segment are only interested in articles covering a certain topic. This is useful information when deciding which product offers or email content to send to each of these customer segments.
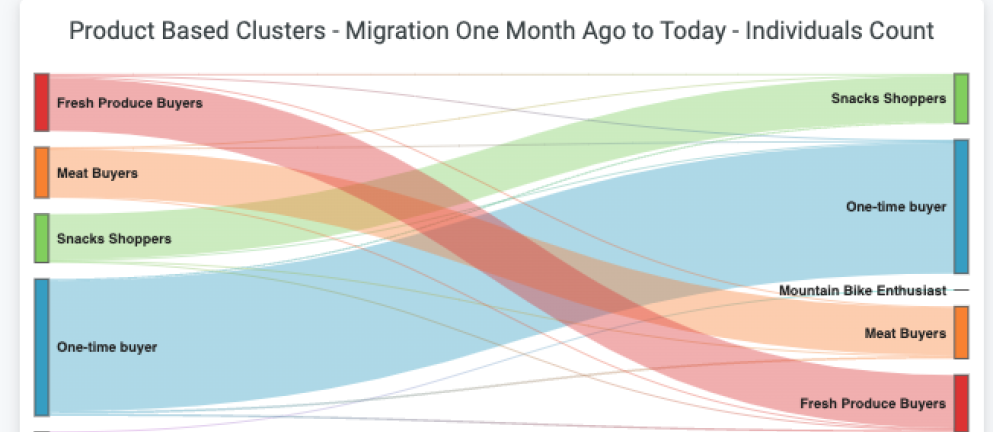
Propensity models
Propensity models are what most people think of when they hear “predictive analytics.” Propensity models make true predictions about a customer’s future behavior. With propensity models, you can truly anticipate a customers’ future behavior.
Model 4: Predicted lifetime value
Algorithms can predict how much a customer will spend with you long before customers themselves realize this. Spend could be a traditional purchase or the likelihood to sign up for a subscription or apply for a financial account. When a customer makes their first purchase, you may know a lot more than just their initial transaction record. You may have email and web engagement data, for example, as well as demographic and geographic information. By comparing a customer to those who came before him or her, you can predict with a high degree of accuracy their future lifetime value. This information is extremely valuable, because it allows you to make value-based marketing decisions. For example, it makes sense to invest more in those acquisition channels and campaigns that produce customers with the highest predicted lifetime value.
Model 5: Predicted share of wallet
With predicted share-of-wallet models, you can estimate what percentage of a person’s category spend you’ve currently met. For example, if a customer spends $100 with you on groceries, is that 10% or 90% of their grocery spending for a given year? Knowing this allows you to see where future revenue potential is within your existing customer base and to design campaigns to capture that revenue.
Model 6: Propensity to engage
A propensity-to-engage model predicts how likely a customer will engage with your communications, such as clicking on an email link, filling out a registration form, or consuming a piece of content. Armed with this information, you might choose not to send an email to a “low likelihood to click” segment when devising an email campaign.
Model 7: Propensity to unsubscribe
A propensity-to-unsubscribe model predicts how likely a customer will unsubscribe from your communications at any time. Knowing this, you can optimize email frequency. For “high likelihood to unsubscribe” segments, you should decrease send frequency, whereas with “low likelihood to unsubscribe” segments, you can increase email send frequency. You can also decide to use different channels (like direct mail or Facebook) to reach out to “high likelihood to unsubscribe” customers.
Model 8: Propensity to convert
The propensity-to-convert model can predict the likelihood that a customer will accept your offer. This model can be used for direct mail campaigns where the cost of marketing is high, for instance. In this case, you only want to send the offers to customers with a high propensity to convert. This model is powerful because you, the end user, define what a “conversion” means, whether that's purchasing a product, booking a care appointment, or applying for a mortgage.
Model 9: Propensity to buy
The propensity-to-buy model tells you which customers are ready to make their purchase, so you can discover who to target. Moreover, once you know who’s ready and who isn’t, you know how aggressive to be with your offer. Those that are likely to buy won't need high discounts — you can stop cannibalizing your margin — while customers who aren’t likely to buy may need a more aggressive offer, thereby bringing you incremental revenue.
Model 10: Propensity to churn
The propensity-to-churn model tells you which active customers are at risk, so you know which high-value, at-risk customers to put on your watch list and reach out.
Propensity models can often be combined to make campaign decisions. For example, you may want to do an aggressive customer win-back campaign for customers who have both a high likelihood to churn and a high predicted lifetime value.
Collaborative filtering
The common marketing term for collaborative filtering models is "recommendations." These recommendation models were made famous by Amazon with its “customer who liked this product, also liked . . .” suggestions. There are different types of recommendations.
Model 11: Up-sell recommendations
Up-sell recommendations are typically made to customers at the time of purchase, such as at the time of online, phone, or in-store check out. You could suggest a higher-end version or a multi-pack of the same product, perhaps at a better price. Up-sell recommendations are typically tied to a specific SKU; every product has suggested products to up-sell to.
Model 12: Cross-sell recommendations
Cross-sell recommendations are also made at the time of purchase. Rather than recommending buying a larger or better version of a specific product, however, cross-sell recommendations suggest other products that are typically bought with a specific item. The recommendation could read: “Customers who bought this also tend to buy . . . ," and you could offer a modest discount if the customer decides to follow your cross-sell bundle. Cross-sell recommendations also tend to be tied to a specific SKU; every product has suggested products to cross sell with it.
Model 13: Next-sell recommendations
Next-sell recommendations are typically made after a customer has already purchased a product from you and could, for example, be included in the confirmation email. The best next-sell recommendations are specific to each customer and take into account more customer data than just their most recent transaction. An example of a next-sell use case was documented in data-driven marketing: A home improvement store found that people who build decks tend to be in the market for a grill shortly thereafter, so a program was established to capitalize on this knowledge.
Model 14: Next-best content
Next-best content models are extremely powerful, especially for businesses that aren’t focused on product. What content should someone consume to drive them to apply for a financial fund? Or book a care appointment? Knowing what content people have an affinity for can be useful in serving them relevant information in the channel they prefer.
The first rule of predictive analytics
Predictive analytics models are great, but they’re ultimately useless unless you can actually tie them to your day-to-day marketing campaigns, which leads me to the first rule of predictive analytics: Always make sure that your predictive analytics platform is directly integrated with your marketing execution systems, such as your email service provider, website, call center, or POS system. It’s better to start with just one model but use it in day-to-day marketing campaigns rather than having 14 models with data that marketers can’t act on.
A customer data platform (CDP) can help you incorporate predictive analytics into your marketing. Learn how implementing a CDP can accelerate your marketing impact.